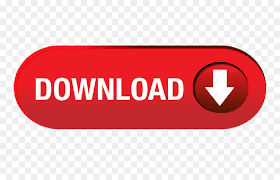
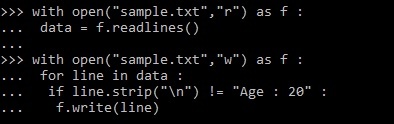
E.g., an ML model is a Transformer that transforms a DataFrame with features into a DataFrame with predictions. A Transformer is an algorithm that can transform one DataFrame into another DataFrame. E.g., a learning algorithm is an Estimator that trains on a DataFrame and produces a model. An Estimator in Spark ML is an algorithm that can be fit on a DataFrame to produce a Transformer. In Spark NLP, all Annotators are either Estimators or Transformers as we see in Spark ML. Please don’t call the ghostbusters, just use the brand new Spark NLP DocumentNormalizer annotator! :Dīut wait, what is an annotator? o.O Let’s see the definition to have an idea. Imagine you aggregate a collection of raw HTML documents you just collected from a given data source with your preferred crawler library and you want to remove all the tags to focus on the tag contents.
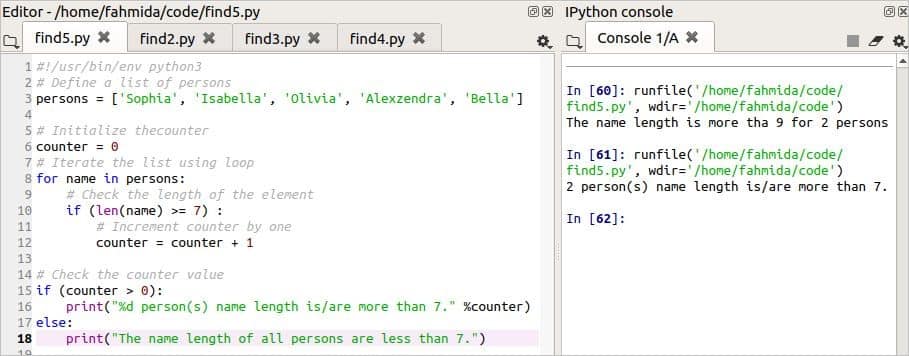
Spark NLP community expressed the need for an annotator capable of directly processing input HTML/XML documents to clean or extract specific contents. Today I’m going to talk about a new annotator that was added in the latest release: the DocumentNormalizer.

This includes new annotators for Google T5 (Text-To-Text Transfer Transformer) and MarianMNT for Neural Machine Translation - with over 646 new pre-trained models and pipelines. support to state-of-the-art Seq2Seq and Text2Text transformers.more accurate, faster, and support up to 375 languages.Some more impressive numbers from the latest 2.7.x release:
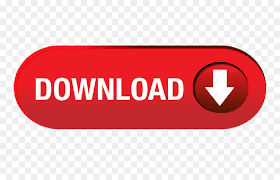